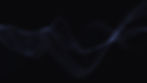
Research
Explore research by The Rohs Lab
1.
Predicting binding specificity in protein-DNA interactions is a challenging task even in the era of AlphaFold. It remains an essential task for understanding gene regulation. Protein-DNA complexes exhibit binding to a selected target site whereas a protein binds with varying degrees of binding specificity to a range of sequences. This is information that is not directly accessible in a single structure. We developed Deep Predictor of Binding Specificity (DeepPBS), a geometric deep-learning model designed to predict binding specificity from protein-DNA structure. The DeepPBS architecture allows investigation of different family-specific recognition patterns. DeepPBS can be applied to predicted structures, molecular simulations, and can aid in the modeling of protein-DNA complexes. DeepPBS is interpretable and can be used to calculate protein heavy-atom importance scores. When aggregated at the protein residue level, these scores conform well with alanine scanning mutagenesis experiments. Beyond predicted binding specificity for biological complexes, we present applications of DeepPBS on in-silico designed proteins targeting specific DNA sequences. DeepPBS offers a foundation for machine-aided protein-DNA interaction studies, guiding experimental choices and synthetic biology, and advancing our understanding of molecular interactions. See for more details: https://www.biorxiv.org/content/10.1101/2023.12.15.571942v1

2.
Rapid advancement in the computational methods of structure-based drug design has led to their widespread adoption as key tools in the early drug development process. Recently, the remarkable growth of available crystal structure data and libraries of commercially available or readily synthesizable molecules have unlocked previously inaccessible regions of chemical space for drug development. Screening-based drug development approaches are limited in their scalability due to computational limits and the sheer scale of drug-like space. A method within the quickly evolving field of artificial intelligence (AI), deep generative modeling, is extending the reach of molecular design beyond classical methods by learning the fundamental intra- and inter-molecular relationships in drug-target systems from existing data. Here in rohs lab, we developed DrugHIVE, a deep structure-based generative model that enables fine-grained control over molecular generation. DrugHIVE is able to accelerate a wide range of common drug design tasks such as de novo generation, molecular optimization, scaffold hopping, linker design, and high throughput pattern replacement. Our method is highly scalable and can be applied to high confidence AlphaFold predicted receptors, extending our ability to generate high quality drug-like molecules to a majority of the unsolved human proteome. See for more details: https://www.biorxiv.org/content/10.1101/2023.12.22.573155v1

3.
Understanding the mechanisms of protein-DNA binding is critical in comprehending gene regulation. Three-dimensional DNA shape plays a key role in these mechanisms. Our method, Deep DNAshape, fundamentally changes the current k-mer based high-throughput prediction of DNA shape features by accurately accounting for the influence of extended flanking regions, without the need for extensive molecular simulations or structural biology experiments. By using the Deep DNAshape method, refined DNA shape features can be predicted for any length and number of DNA sequences in a high-throughput manner, providing a deeper understanding of the effects of flanking regions on DNA shape in a target region of a sequence. Deep DNAshape method provides access to the influence of distant flanking regions on a region of interest. Our findings reveal that DNA shape readout mechanisms of a core target are quantitatively affected by flanking regions, including extended flanking regions, providing valuable insights into the detailed structural readout mechanisms of protein-DNA binding. Furthermore, when incorporated in machine learning models, the features generated by Deep DNAshape improve the model prediction accuracy. Collectively, Deep DNAshape can serve as a versatile and powerful tool for diverse DNA structure-related studies. See for more details: https://www.biorxiv.org/content/10.1101/2023.10.22.563383v1

4.
Three-dimensional structure of RNA molecules encompasses a wide variety of complicated topologies and chemical interactions. Significant amount of effort has been spent to generate RNA secondary structure visualizations. However, these layouts are often non-representative of the true geometry of the tertiary structure. In other cases, widely used programs are no longer user friendly or state-of-the-art given today’s visualization, computation, and file formatting standards. There is an urgent need for a modern two-dimensional mapping and visualization of RNA structures which more correcly reflects its three-dimensional geometry. We developed the RNAscape web server which produces modern, customizable, and publication quality images of two-dimensional RNA visualization using a redesigned structural mapping algorithm. In addition to creating this visualization, the RNAscape web server allows users to interact with the visualization and modify it through advanced settings as desired. RNAscape is applicable to DNA and RNA/DNA hybrid structures. It can be used to decipher and quantitatively describe nucleic acid structure and interactions, which in turn are the basis for machine learning and artificial intelligence (AI) methodologies and applications in structural and synthetic biology. See for more details: https://rohslab.usc.edu/rnascape/

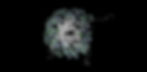